
Tignis simplifies adding ML to advanced process control manufacturing systems
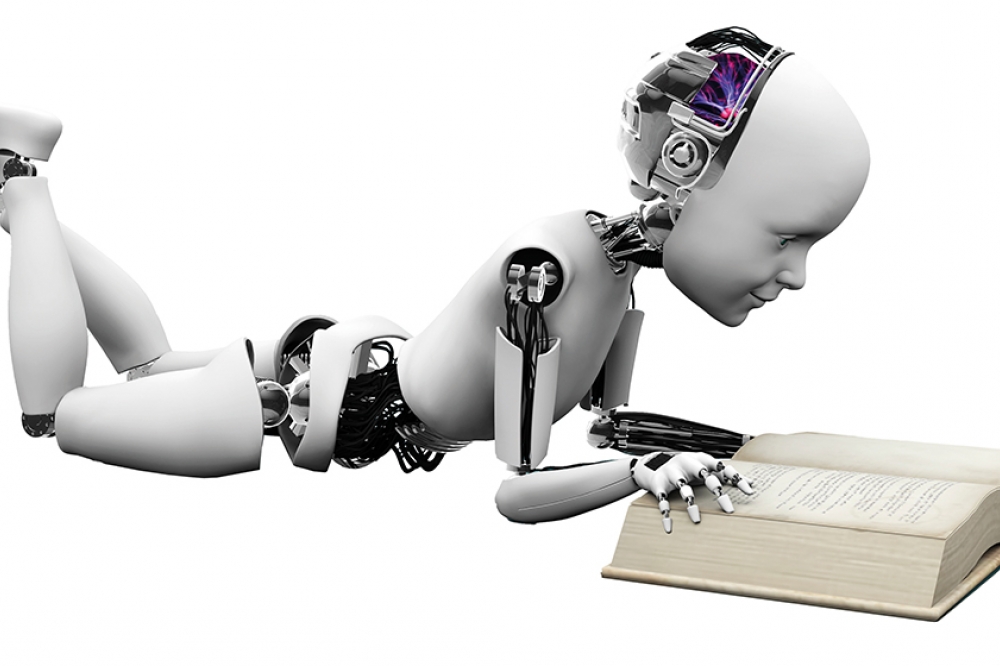
With the continual buzz around artificial intelligence (AI) and machine learning (ML), one might think it relatively easy to improve manufacturing output by adding a ‘bit’ of AI/ML to one’s IC recipe. But the reality has been far more complicated. Enter the AI process control experts at Tignis who believe they have a new solution that could make ML-driven APC much more accessible and cost-effective.
IMAGINE a small semiconductor manufacturer that wants to add a machine learning-aided application to improve its fabrication process flow. The company is about to discover that besides their process engineer, adding ML capabilities is a multidisciplinary approach with no off-the-shelf solutions that are fast, highly adaptable or easy to implement. According to Tignis, a Seattle-based AI-powered process control company, that paradigm is about to shift.
Tignis has announced its new AI/machine learning analytics tool set that it calls PAICe, which it describes as a solution for companies to add the benefits of AI/ML APC without the substantial investments of time and money that has previously been the norm. Company co-founder and CEO Jon Herlocker told Silicon Semiconductor in a prerelease interview that PAICe is designed to put the power of machine learning into the hands of non-data scientists. This next-generation technology helps manufacturers achieve process improvements not previously possible with today’s advanced process control (APC) approaches including the ability to utilize surrogate machine learning models created by Tignis that are more accurate and up to one million times faster than physics-based simulations. This performance enhancement can directly lead to faster production, better quality control and faster time to market.
“In creating PAICe the basic idea is to empower the process engineer. This is the opposite approach that some solutions providers offer today that essentially take a ‘magic black box’ approach that does what you want, but you have no idea what goes on inside the box and as a customer, you are totally dependent on another company’s technology; you give up control. Our approach—using what we call our digital twin query language, or DTQL, is to be able in minutes to build models without even one course in data programming. The basic system is ideal for at least 80 percent of use cases, and we can offer our own programmers for unique situations that need further optimization,” he explained.
According to Tignis, their new DTQL is the first language designed specifically to build machine analytics on digital twins. Through DTQL, Tignis says its PAICe product suite significantly removes the obstacles that have prevented engineers from leveraging all the historical data they have collected to help them make better decisions; the new solution enables process and reliability engineers to convert their deep subject matter knowledge into hundreds of machine learning based predictive models that are easily managed across thousands of diverse physical assets – all without having to become a data-scientist.
The PAICe product suite accelerates the ability to build, validate and deploy machine learning enabled solutions in manufacturing and process industries, with an initial focus on semiconductor manufacturing, oil and gas processing, and energy. It is the latest venture by Jon Herlocker, a serial entrepreneur and deep technologist with a track record of founding and building successful startups, as well as a former VP and CTO at VMware, a $12 billion dollar a year virtual infrastructure management company. Tignis is funded and advised by industry leaders such as software executive Paul Maritz, who is an investor and member of Tignis’ board of directors. Maritz was CEO at VMWare, and at Microsoft he was a member of the top executive management team. Harel Kodesh, former CTO of GE Digital, is also an investor.
“The PAICe product suite puts machine learning in the hands of people that have never been able to use it before,” said Herlocker. “This is important because machine learning-based control algorithms not only outperform classic feedback or feedforward advanced process control applications, they continuously learn from new process data, reducing the need to retune controls and improve over time. With the PAICe product suite, many more manufacturers will now be able to take advantage of the benefits of machine learning in modern manufacturing and process control by increasing process quality, throughput and yield.”
The PAICe product suite enables machine learning for more than just predictive maintenance – it enables it for process optimization and its implementation directly into process control loops. It is able to run machine learning-based simulations one million times faster than legacy physics-based simulations, allowing manufacturers to have real time feedback control in places that were not possible in the past such as real-time optimization. Key features of the suite include:
PAICe Builder, a machine learning analytics tool easy enough for anyone to use. It provides simple connectivity to OSIsoft PI data historian and other data sources, and is available in both downloadable or cloud versions, allowing users to do analytics anywhere.
PAICe Monitor, which allows customers to easily deploy their analytics to private or public cloud infrastructure and thousands of assets with one click (including web APIs to ingest and send data to and from data historians). It offers a scalable cloud infrastructure to build analytics as needed; the Tignis managed infrastructure means customers only pay for the resources they need.
PAICe Maker, which deploys and manages machine learning based control algorithms that improve over time with more data. Machine learning models can compute control variables at speeds up to one million times faster than legacy physics-based simulations, allowing real time computation of control. Hybrid on premise and cloud architecture ensures low latency for control but the best possible model training and learning in the cloud.
Through the company’s extensive beta test program prior to launch, the PAICe product suite is in use by a number industrial clients spanning the oil and gas, semiconductor and energy industries. Some notable users of the product suite include Tokyo Electron (TEL), Synopsys, Etairon, and Optimum Energy. The product suite is now available directly from Tignis; visit www.tignis.com for details.