Chasing the world’s first Brain-on-Chip AI computer
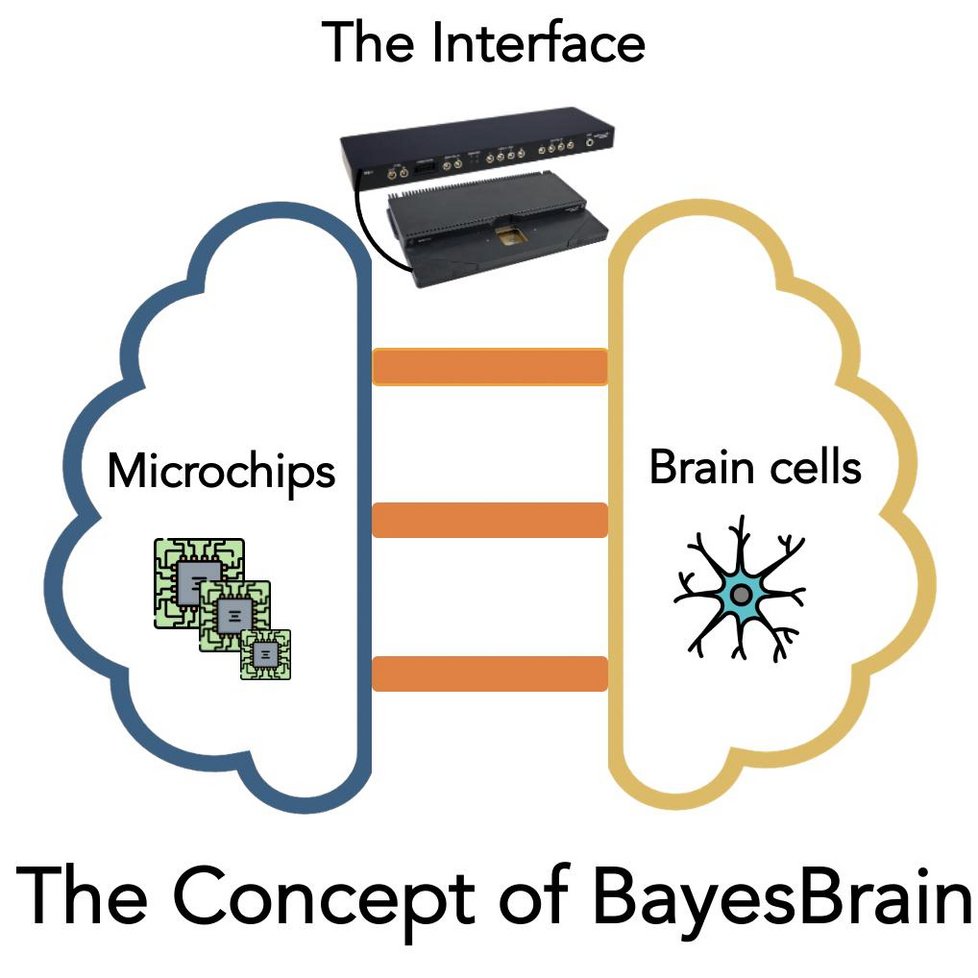
New project BayesBrain aims to build the world’s first computer that combines brain cells and silicon microchips.
Besides being the most intelligent computing system ever, the brain is also very energy efficient. It uses orders of magnitude less energy than traditional computers, which makes it attractive for future sustainable computing hardware. TU/e researchers led by Regina Luttge and Bert de Vries recognize the revolutionary potential for a hybrid computer consisting of brain cells and silicon microchips, which could be used to solve real world problems such as low-power wearables, IoT devices, and advanced controllers for AI technologies.
The office walls of TU/e researchers Regina Luttge (Mechanical Engineering) and Bert de Vries (Electrical Engineering) are adorned by art. Hanging over Luttge’s desk is a colorful piece that she painted herself, while in De Vries’ office there’s a painting of a man with a mathematical formula in the top-left hand corner.
Besides their contrasting office art, the pair also work in different fields. Luttge’s research focuses on growing brain cells in so-called Brain-on-Chip devices, while De Vries looks at computational neuroscience, signal processing, and machine learning.
Yet, the pair have joined forces for a high-risk high-gain project at TU/e with the ambitious goal of building the world’s first Brain-on-Chip AI computer that can solve problems in real-time and in a low-energy manner.
In other words, the researchers want to build a device where brain cells work with a silicon-based computer to enhance ultra-low power wearables, IoT devices, or controllers with AI technology in the future.
And the project’s name? The BayesBrain project.
The Bayes part of the project name comes from the English 18th century statistician Thomas Bayes,” points out De Vries. “And it just so happens that the man in the painting in my office is Thomas Bayes with the equation above him known as Bayes’ theorem.”
Bayes’ theorem calculates the probability of one event happening based on the probabilities associated with another event. Bayes work and name have subsequently been used in Bayesian machine learning, a field that De Vries has been working in for a number of years.
The “Brain” part of the project name comes from the human brain. But why do the researchers want to create an AI computer with brains cells working in tandem with silicon-based microprocessors?
“The unescapable truth is that our modern computers consume too much power, but brain cells use orders of magnitude less power,” explains Luttge. “And with our computing demands increasing, we need more sustainable computing. Studying brain cells in this context offer a potential solution.”
Energy matters
Combining a silicon-based computer with brain cells in BayesBrain is definitely not a publicity stunt. If this approach can be scaled-up, it could have far-reaching consequences for machine learning in the future.
“Right now, the training of some artificial neural networks by DeepMind and Google incurs huge financial and energy costs. On the other hand, the brain uses many orders of magnitude less power to function, but we can’t achieve the same orders of magnitude change with current computers. To attain this, we need a paradigm switch, and hybrid computing involving brain cells could be the answer,” says De Vries.
In a reactive system such as the brain, cells never spend any energy on passing messages to other brain cells unless it improves the accuracy of the computational task. In contrast, in a conventional algorithmic approach to computation, messages are passed regardless, even when they don’t improve the accuracy of the result, and this affects energy efficiency dramatically.
Balancing pendulums
BayesBrain is aiming to do something that sounds like it’s from science fiction. Besides Luttge and De Vries, the rest of the BayesBrain team is made up of TU/e researchers Burcu Cumuscu-Sefunc (Biomedical Engineering) and Wouter Kouw (Electrical Engineering), as well as Robert Peharz (Graz University of Technology, formerly TU/e). So, what are the team planning to do?
On the brain cells side, about 1,000 brain cells will be placed in a so-called microfluidic Brain-on-Chip device. There the cells will form tiny neural circuits thanks to a compartmentalized microfluidic systems. To keep the cells alive, they’ll be supplied with water, nutrients, and an incubating environment (temperature, atmosphere, etc.) that they need to stay healthy over time.
“The cells should form sufficiently matured neural networks after about three weeks. Once the tiny neural networks form and reform (a phenomenon called neural plasticity), the brain cells will be ready to ‘talk’ to the silicon-based computer,” says Luttge.
For the Bayesian part, the team will use a silicon-based computer that solves problems based on Bayesian inference. And it’s from this purely silicon-based device that the researchers will start the project.
“We’ll start with a 100% silicon-based Bayesian computer and use it to solve a simple real-time control task, such as the inverted pendulum problem,” says De Vries.
The inverted pendulum problem will serve as the validation problem for the devices designed and constructed as part of BayesBrain. It’s equivalent to balancing a stick on a moving platform, and is a classic problem used in reinforcement learning, a machine learning training method which rewards favored outcomes and penalizes unfavored ones.
“This problem is not new, and we know that it can be solved with a silicon-based computer,” notes Luttge. “But if we can solve this problem using a system that’s made up of a silicon-based microchip and a Brain-on-Chip device containing real brain cells, it will be extraordinary! That’s because this problem has never been solved using a computer made up of brain cells and microchips.”
Interface eagerness
The researchers have a solid plan to solve the validation problem – the aforementioned inverted pendulum problem – using the hybrid computing device.
“Once the silicon side of the device is balancing the inverted pendulum, we’ll start to transfer some of the computational load from the silicon side to the brain cells side of the device,” says De Vries. “We’ll start by replacing a small part of the load at first, and slowly replace more and more of the silicon part with brain cells.”
This approach is dependent on the development of an interface that allows the brain cells to talk to the silicon-based computer, and vice-versa.
“The communication interface is perhaps the greatest problem to be solved in this project. Without it, the brain cells won’t be able to share the computational load,” adds Luttge.
In effect, the research team need to create a device that allows each side to “hack” the other side. Bert de Vries: “It’s a collaboration between two agents that are hacking each other. And we’re the facilitators for this hacking.”
Chasing a first
Although the team will just use the hybrid computer to control an inverted pendulum in the project, there are plenty of real-world situations where this hybrid device could be used.
Think of air traffic control at an airport where dozens of aircraft might approach and leave at any one time. Or directing multiple ships as they approach a busy harbor.
“The main thing is that this is a fresh start with potential for revolutionary changes. We plan to use the most intelligent system ever – the brain – as a component in what could be the world’s most energy-efficient computing device,” De Vries notes.
“I have to admit that I’m excited to get started, excited by the possibilities,” adds Luttge.
Speaking the same language
With any interdisciplinary collaboration, it’s important that the researchers speak the same language, and this project is a prime example. And De Vries and Luttge both admit it’s taking time for them to adjust to each other’s vocabulary.
“It’s hard for us given that we’ve spent our careers speaking in a particular way,” notes De Vries.
Luttge agrees: “It’s great to be working on such an innovative project, but we need to make sure that we understand each other’s language as well as the technical aspects of what we do.”
Both are quick to point out that the two PhD researchers who will join the team will have it slightly easier. “They’ll learn the language of both worlds during their training, so it’ll be a little easier for them to adjust to the different concepts and definitions from our fields,” says Luttge.
Importantly, there is understanding between the researchers. “We are 100% forgiving with regards to how we talk about this research,” De Vries says with a smile.
And who knows – if Luttge, De Vries, and their collaborators are successful in their pursuit of the world’s first Brain-on-Chip AI computer, future brain and machine learning researchers might have artistic renderings of the BayesBrain research team on the walls of their offices.
BayesBrain funding information
BayesBrain is funded by the EAISI (Eindhoven Artificial Intelligence Systems Institute) Exploratory Multidisciplinary AI Research (EMDAIR) call. The funding covers the hiring of two PhD researchers to work on the project.