CEA-Leti reports Neural Network breakthrough
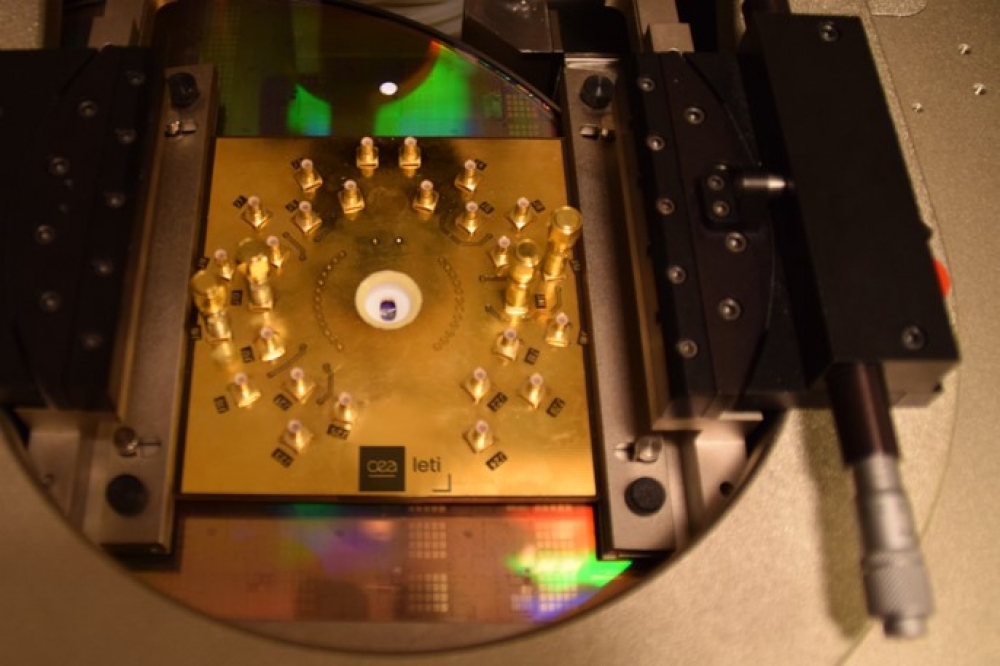
A team comprising CEA-Leti, CEA-List and two CNRS laboratories has published a new paper in Nature Communications presenting the first complete memristor-based Bayesian neural network implementation for a real-world task—classifying types of arrhythmia recordings with precise aleatoric and epistemic uncertainty.
Considering medical-diagnosis and other safety-critical, sensory-processing applications that require accurate decisions based on a small amount of noisy input data, the study notes that while Bayesian neural networks excel at such tasks because they provide predictive uncertainty assessment, their probabilistic nature requires increased use of energy and computation. The increase is caused by the fact that implementing the networks in hardware requires a random number generator to store the probability distributions, i.e. synaptic weights.
“Our paper presents, for the first time, a complete hardware implementation of a Bayesian neural network utilizing the intrinsic variability of memristors to store these probability distributions,” said Elisa Vianello, CEA-Leti chief scientist and co-author of the paper, “Bringing Uncertainty Quantification to the Extreme-Edge with Memristor-Based Bayesian Neural Networks”. “We exploited the intrinsic variability of memristors to store these probability distributions, instead of using random number generators.”
A second major challenge was that performing inference in the team’s approach requires massive parallel multiply-and-accumulate (MAC) operations.
“These operations are power-hungry when carried out on CMOS-based ASICs and field-programmable gate arrays, due to the shuttling of data between processor and memory,” Vianello said. “In our solution, we use crossbars of memristors that naturally implement the multiplication between the input voltage and the probabilistic synaptic weight through Ohm’s law, and the accumulation through Kirchhoff’s current law, to significantly lower power consumption.”
Reconciling Memristors and Bayesian Neural Networks
Co-author Damien Querlioz, a scientist associated with the University of Paris-Saclay, the French National Center for Scientific Research (CNRS) and the Center of Nanosciences and Nanotechnologies, said the team also had to reconcile the nature of memristors, whose statistical effects adhere to the laws of device physics, with Bayesian neural networks, in which these effects can take arbitrary shapes.
“This work overcomes that challenge with a new training algorithm – variational inference augmented by a ‘technological loss’ – that accommodates device non-idealities during the learning phase,” he said. “Our approach enables the Bayesian neural network to be compatible with the imperfections of our memristors.”
Uncertainty quantification involves the network's ability to identify unknown situations out-of-distributions.
If a traditional neural network trained to recognize cats and dogs is presented with an image of a giraffe, it “confidently misclassifies” it as a cat or a dog, Vianello said. “In contrast, a Bayesian neural network would respond, ‘I am not entirely sure what this is because I have never seen it.’ While this example is lighthearted, in critical environments like medical diagnosis, where incorrect predictions can have severe consequences, this uncertainty-capturing ability becomes crucial.”
This capability arises from the fact that synaptic values in Bayesian neural networks are not precise values, as in traditional neural networks, but rather probability distributions. Consequently, the output is also a probability distribution, providing information about its “certainty”.