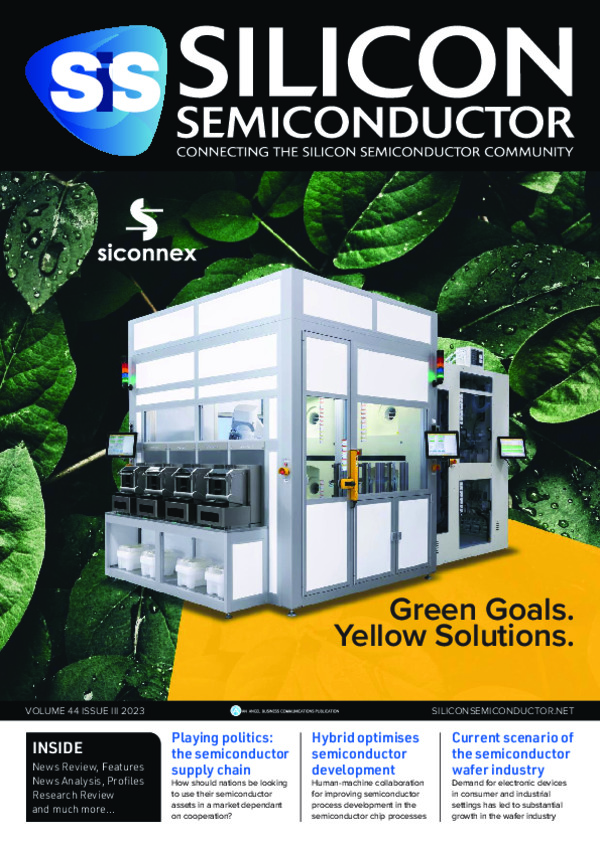
A hybrid approach optimises semiconductor process development
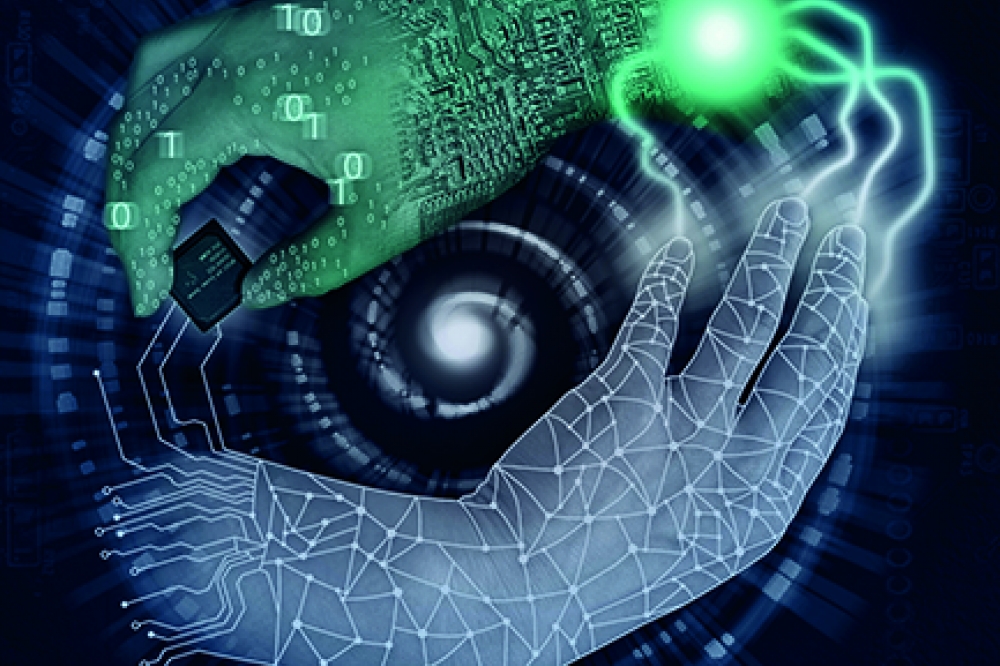
Keren Kanarik, Technical Managing Director in the Office of the CTO at Lam Research, and co-author of the Nature-published article: Human-machine collaboration for improving semiconductor process development, discusses with SiS editor, Philip Alsop, the organisation’s research into what combination of human and artificial intelligence might best decrease the cost of developing complex semiconductor chip processes.
PA: Lam Research recently published a paper which, in simple terms, was looking at AI and humans in terms of designing process engineering for semiconductor applications. Perhaps you can just give us a flavour of the paper and then we’ll drill down into what actually went on in terms of from start to finish?
KK: Our paper addresses a challenge, and one of the bottlenecks, really, of chip making, and that’s the process engineering, the cost and time of process engineering, which is developing the processes that make the chip. And for the past 50 years, that’s been done by humans, but it’s taking longer and it’s costing more, especially as we’re moving to EUV. And so the question that we wanted to answer in this paper is whether AI could help accelerate those efforts and reduce the cost of process engineering. So we went about trying to answer this question by running a competition, basically a game, to benchmark the different AI and different human approaches.
PA: Intuitively, one would imagine, just the way that AI is taking over the world, that AI would automatically be better than humans because it can just process more information more quickly. But I’m suspecting that it may not be as simple as that. Is that correct?
KK: Yeah, I like this question a lot. So, on one hand, as you say, intuitively, you think that AI should be able to handle this process engineering, tuning a process. On the other hand, process engineers like me, we know how much we rely on experience to do the process engineering. And so really, beforehand, we were sceptical of what AI could help or not help. So what the issue comes down to is that we’re dealing with little data due to the high cost of data acquisition. And what that means is that we’re not just asking the AI to develop a process, we’re asking it to do so at the lowest possible cost. And it’s that cost constraint from the little data. That’s what really makes this problem interesting. Because really it wasn’t clear from the beginning who was going to win.
PA: Okay, so that was the background to how you decided to investigate AI versus human process engineering. Did you have any preconceptions at all? I know it’s very difficult when you start with studies. Often, even if you say you don’t, you have some kind of idea of where it might go. Did you have any thoughts or it was literally a blank piece of paper and you were going to be interested to see where it went?
KK: Well, I came about this very objectively. The data scientists were coming to me and claiming that AI could help reduce the cost and kind of solve this problem. But as I mentioned, I was very sceptical. So that’s where we came up with this idea because I didn’t really know whether to believe them or not. So that’s where we came up with this idea of doing the competition.
PA: And in terms of the competitions, I understand that you developed a virtual process game. So can you talk us through it? Sounds exciting. What was involved in doing it and what was the thinking behind what you wanted to achieve with it?
KK: I wanted to give the data scientists a chance to show what AI could do. And so originally I thought to run this game, this competition, on real wafers. And I brought up this idea to my boss at the time, Rick Gottscho. He’s a co author on the paper, and he’s the one that suggested that we do it virtually so that we wouldn’t have to deal with the noise and the variability of real wafers, which could maybe muddy the results. So once we thought of doing it virtually, the advantages of doing it virtually became pretty apparent. We were able to run as many games as we wanted over and over again on the same process, apples to apples comparison. Actually, we ended up running hundreds to thousands of games, which really helped with the statistics for the AI performance. And then how we ran this competition. So like in a real lab, the participants would pick the recipe conditions and then it’d be run through a simulator. We’d give back the results. And so effectively, at the end of the day, it had the look and feel of a real demo.
PA: Okay, in terms of the participants, they were the humans and then the AI, the computers. So first up, it would be good to understand how the humans came out in terms of how they performed in the game?
KK: So we found that the humans were able to make cost effective decisions with the little data. And if you think about it, this makes sense because apparently humans have evolved to make decisions without all the information. For example, what college do we go to? What house do we buy? What are we going to eat for lunch? And we call this intuition. But in process engineering, the way I think of this is the engineers have formed some sort of mental map of how the space looks like and it helps us navigate through the processes. So in contrast though, for the humans, we observe that in the fine tuning stage, it didn’t matter how much experience or whether they had a mental map in their head or not, whether they were Senior Engineer, junior Engineer, HR, it was almost as if they were shooting in the dark. They would change one or two parameters at a time and almost it appeared almost randomly.
PA: And in terms of the contrast for the, for the computer participants, where did they fall down or and where did they maybe shine?
KK: Okay, so the computers, fortunately, we found what I would call the opposite behaviour. So their advantage was in the fine tuning stage. And there the computers, they’re able to move all of the parameters at once in such ways to find winning recipes rather quickly, as long as they were given guidance from the human where to look. In contrast, flipping it around, when the process space was too large. So at the beginning of development, they just got lost. We could see they were not making good progress towards the target. It makes sense that they lack the experience, they don’t have the mental model, but they also don’t have a computer map model to rule out maybe unlikely spaces or promising regimes. So the way I think of it, they didn’t have this map to follow.
PA: But as you say, once they’d been given a reasonable size of accurate data, they were better, and I guess faster in particular at coming up with possible fine-tuning options and then coming up with the winning recipe. Is that right?
KK: Yeah, they were able to. But, fortunately and reassuringly to us, we found that the humans are essential for that to happen. So they weren’t able to do it completely on their own. Actually, the algorithms they could find, like I mentioned before, they could find the target on their own. So I just want to clarify that. But again, they couldn’t do so at a low cost, which was the essence of the competition and the little data problem that we talked about. So in the end, I kind of think of the data scientists were right and they were wrong. So AI could help, but only after given guidance from the experts.
PA: Okay. And then I believe that the next step. And fairly logical, based on what you’ve explained about the relative strengths and weaknesses of the humans and the AI computers, you came up with a hybrid game or scenario where you describe it as humans first, computers last. So talk us through what you did at that stage.
KK: Okay, so we would give the computer and when I say computer, the computer or the machine or the AI, so we’d give the computer data from the human up until what we called the transfer point, along with a constrained space for them to look. And then the human effectively would step away. You can picture the human went out for coffee. By the way, I want to mention that it might look obvious in retrospect that we tried this hybrid approach of human first and computer last. But if you really think about it, we could have tried the other way around. We could have done computer first and human last, if we had assumed instead that the computer would do better at the beginning of development when the number of options is the highest. Right. But as we saw and with the concepts that we’ve been talking about, we chose the other way around because we could see pretty clearly that the algorithms with their trajectories, they were struggling early in development.
PA: And just to throw in a question, did you run any of the scenarios where money was no object and did that just to see whether in that scenario, if computers, if you could spend millions or billions and billions on computers, they’d eventually come up with the right result?
KK: A lot of the trajectories we truncated just because of time and cost, of just why keep running? But we did allow one of the trajectories to go for as long as it wanted, like you said, as if there was no cost involved. It took don’t remember the exact number, but about $750,000 in order to find the process on its own. And that is relative to the expert benchmark, which was $105,000.
PA: In terms of what you discovered, whether we’re sort of re-emphasizing, that experienced humans were very important for the overall success of the process engineering, and the findings very much supported that, is that correct?
KK: Yes. So, again, I do want to emphasise that we did find that the humans are essential. And again, being a former process engineer myself, which is why I ran this competition and was interested in this, that was very reassuring in terms of not only did we find them essential. But as you’re getting at, the more experience seemed to be better. And the way you can see this, I think really clearly in the paper there’s this figure three and extended data, and where we teamed up the computer algorithm with each of the process engineers and one of the inexperienced players, which you can assume is someone from HR department, let’s say, and we found pretty clearly there that when you team up the computer with the more senior engineers, the overall costs were reduced. Now, there was kind of one exception there. There was one junior engineer that when they teamed up with the computer, did as well as the senior engineers. And when the reviewers were going through this paper, they joked that we should promote that junior engineer. She’s doing quite well!
PA: And in terms of the contrasting finding, you discovered or confirmed that uneducated computers are not very suited to the task. They are only really useful when they have access to, shall we say, the refined data. Is that fair to say?
KK: Yeah, that’s essentially so. Again, you saw with the example where we let the computer go until it wanted to and it cost 700 something thousand dollars, right? So the AI could, like the data scientists claim it could find the target. But again, the essence of this was could it find it at lower cost? And so in that sense, the data scientists, again, they were right and they were wrong. The computer could find the target, but it needs the guidance from the expert. And I think what this does, the results really highlight the importance of domain knowledge and experience, specifically in these little data type of problems, especially in our process engineering little data problem.
PA: In terms of bringing that work together and optimising the chosen, if you like, or the preferred hybrid strategy. The crucial bit, and I don’t know again, whether the game was able to reveal that, is at what point the humans stop, hand over their expertise and let the computer, the algorithms, take over to finish the process. Was that easy to identify?
KK: Yeah, that’s a good point. And when you’re doing this hybrid strategy, how do you know when to switch over from the human to the computer? And the simple answer is don’t do it too early and don’t do it too late. But how do you find that point? So I would say if you wanted the absolute lowest cost, there should be this optimal point that we identified at the bottom of the V, but we identified it in retrospect, right? So if you don’t know ahead of time where that point is going to be, and we show that it depends on the process engineer, it depends on the computer algorithm, it will depend on the process. You may not always know that. That said, I think what we also finding is that you don’t need the transition to be exactly right to still get the cost benefits of this approach. There was quite a wide range of that V of transition points that would still provide cost savings over doing a human alone.
PA: And in terms of perhaps slightly stepping back a bit for more general findings, although this combined, this hybrid solution is the preferred one, you identified, I think, or you’re suggesting that there might be some cultural issues with that sort of combination. So did that come out in the test or is this just anecdotal this is what you think might be the problems if this was enacted in the real world?
KK: Well, first let me explain because I do, I really like that aspect of the paper hunt, this people, cultural side. Because what’s happening here is we’re proposing to partner humans with machines and that’s foundationally changing the way processes are being developed since the last 50 years and not making that change. It’s not easy to make that change. So really what we are seeing and we’re watching the computers, we’re watching the humans, that the AI does not behave like the humans. And there’s what I’ll call potential trust issues. To me, the really most clear example is that we found, and this is some in the extended data, we found that when it was handed over to the computer, the first thing that happened is often the computer would make these unusual, maybe non-traditional moves, moving all the parameters at once. And that means that the human needs to have faith that even though the computer is exploring, eventually it will hit target, preferably faster than the human would have. And there’s no guarantee that that’s actually going to happen.
And so I think of it like I looked at my phone the other day on the weather app and it had been predicting the rain pretty nicely. And then one day I look outside and it’s raining and my phone is not saying that it’s raining. And that doubt, that wonder is the AI working or not? Sometimes when there’s no guarantee that there’s some issues there that are known. We put some references, I did some references there. It’s known that it’s difficult to sometimes work with computers that way.
PA: And did you at an even more basic level, did you get some of your more experienced process engineers just kind of say, well, I’ve got a whole career’s worth of knowledge and I don’t buy the idea that the AI can actually improve what I’m capable of doing. Was there a level of scepticism?
KK: Yeah, that’s really interesting. So I would say overall, the people, the engineers, they are actually really open to this AI help in the way in this approach that we’re showing because we identified these two regimes, the rough tuning and the fine-tuning and really this confidence of the process engineer and it’s really in the rough tuning phase. That’s where they do well. That’s where they see a lot of progress. The truth is that when they get to the fine-tuning phase, there is some frustration there, and they are more than happy to have the computer go and tidy up their work. It’s like they do that 80:20 rule. They do 80% of the process, and they are happy to hand off that 20% to the computer.
PA: In terms of where we are now with AI’s usefulness or not with semiconductor process engineering, it would be good to understand where you think we are. But also talking to yourself. What is Lam Research currently doing in terms of maybe, as a result of this research, is AI becoming more important to you -where we are with AI and where yourselves in particular?
KK: Yeah, that’s good. So let me step back one moment and just explain to the audience why Lam is even interested in this study and in process engineering at all. We’re in an equipment supplier, and we make the equipment that makes the chips, but we need to show our customers, the chip makers, that these processes work, that our equipment is capable of doing that. And so we have a whole bunch of process engineers in the labs working on this, and that’s why we’re at the front line. So that’s why Lam’s interested in it now, and that’s why we did this study. In terms of using AI to help that process engineering, it’s important to Lam, but really it’s important to the whole industry. We just happen to be at the front lines using this AI. We believe this is in its infancy. We’re just getting started. We believe this paper is representing what we see as the beginning of the use of AI in process engineering.
I also want to give just a little context, perhaps for your audience. When you build a chip, you first have to design it, and then you go in and physically build it. The designing part that has already been computer aided and even using AI for decades, actually, at least computer aided - the design part. But just because you can design a chip doesn’t mean you can make it, right? So this paper really is focusing on the making part, and that process engineering, developing the process to physically make the chip. And that, I believe we are just getting started. And that’s what we believe this paper is representing.
PA: In terms of you said getting started, the obvious question might be, where do you think and I know it’s a bit of a sort of crystal ball gazing, but where do you think AI might get to? And I’m wondering, we mentioned the little data problem. Is it just a question that if you can run machine learning algorithms, for example, and just absorb vast amounts of information and real-world examples, and once all that data has been absorbed, then AI takes over. Or because there are, as I understand it, so many variables in every time you’re designing a process to manufacture a chip, that it’s almost impossible that a computer will ever be able to contemplate all the different nuances. And we’re back to a human’s experience where they will just instinctively know what to do, where a computer won’t be able to learn what to do?
KK: Yeah, I think you’re actually hitting on something important here that’s been brought up and we’ve debated it ourselves. So I would say this - I’ll give you a couple ideas that we’ve thought through in this debate. So you’re asking if we had all the historical data in the world, all the processes out there, would that even help? Right. The thing is that even if you had all historical data, it doesn’t mean you have enough data in the tiny little corner of the process space that you have of interest. And I’m not sure that everyone realises this, but every process is different in some way. It’s a different stack or it’s a different tool to run on. And so, for example, if you ran the exact same process recipe three years ago and then you run it today, you’ll likely not get the same exact result. Because what’s happening is there’s all these latent you mentioned lots of variables. There’s latent variables, there’s hidden variables. And so we believe that at the end of the day, even as much as the AI will help, that there will always be a little data problem, to some extent at least, and in the limit of tight tolerances and that the data is costly.
PA: And presumably the industry is moving so fast as well, so we’ve got more exotic materials being introduced and other innovations. So, again, that’s a problem because again, when that starts happening, then the AI is back to square one. It’s got no reference information, it’s back to human. If I understand it, you think AI has more of a role to play, but it’s never going to be able to replace that human experience because it just will never be able to absorb the required data sets. Is that right?
KK: So I would say that the algorithms I think there’s relative to what we published in the paper, I do think there’s definitely room for improvement. Definitely there’s some more teaching you can do to the AI, but I do agree with what you’re saying, is that first of all, there’s a bunch of new processes with new materials, but I would even go so back as old processes. You think you’ve done a mask, open etch before and you think you’ve done it for the past 50 years and you get a new one and it’s almost like you have to do it again. Although you can transfer some of the humans, transfer some of their experience, but then again, you have to still do some amount of process engineering to dial it into whatever new stack or new tool it happens to be on next. So somehow we still have to do it over and over and over again and so will the computers.
PA: In terms of the hybrid, the human first, computer last, it would appear to be the main benefit is financial? You can hit the object target for the optimised cost if you like or the lease cost, whatever. Is that the main benefit? And did you compare the costs of doing human only and computer? Well, we found out the computers only ended up costing an astronomical amount. But were there other benefits that accrued alongside just the purely financial one?
KK: Yes, in the paper we did focus on the financial one, which is the cost reduction, the cost savings. But along with that there is a really important benefit which is the time reduction that goes along with that cost reduction and with how fast this chip industry is evolving and changing that, speeding up that cost, I mean that time reduction to innovation effectively that’s really important as well. You might even argue more important.
PA: Were you able to put vague percentages or numbers on either the cost optimization, how much you might be able to save or in the time?
KK: That’s good. Off the top of my head, I would say if you reduce the cost by half, you might reduce the time by half because you’re doing that many fewer. We did have a cost function that included number of experiments and the batch of experiments but roughly speaking you would translate that to time.
PA: In terms of moving from a virtual process game to real world, is that the logical next step? Is that something you’re looking at or does virtual give you all that you need - thoughts as to what’s the next step I guess having done in the virtual process game?
KK: Yes, so actually this is already happening in the real world. We are using this AI in the process engineering with real processes at Lam with good results. And that’s partly when you asked the question before about how the humans were accepting. They are excited and for me it’s exciting to see it working. I would still call it infant stages, but it is being used.
PA: I know you’ve obviously published the paper, so it’s in the public domain, but have you worked with any of your key customers, partners to share this knowledge and had any feedback from them as to how confident they are and that what you’re doing is going to make a difference for them, chip manufacturers and stuff? Or is it too early to be doing that work?
KK: Well, I will say we have been talking to customers and they also seem to be quite excited about this work.
PA: In terms of the future. I guess one other variable I didn’t ask about is particularly, I suppose, to do with cost, because quantum computing is on the horizon. And I’m just wondering, might there be a future where the processing power is improved so astronomically, and the price of it has come down correspondingly? That what you’ve discovered in this paper might be somewhat challenged because the financial equations changed or because of the problems we’ve outlined just absorbing all the data sets, or lack of them, that computers will always struggle? So there will be this hybrid solution that always wins out.
KK: Well, I think anything that a computer can go faster and more powerful will help if we’re using computers. But I think really the main challenge for the computers is kind of a little bit what you’ve alluded to is, can we improve them from what we’ve already published that they can do? How much room for improvement is there in these algorithms to, for example, we mentioned in the paper some future directions where perhaps we could encode domain knowledge to reduce the costs even further. So how far can that go? I think that’s what I’m looking for from the direction of computers in this problem.
PA: Just as a matter of interest, do you have any specific roadmap or time frame as to various stages of this? Or is it very much you’re doing the research and as and when something interesting, important crops up, then that will be taken out and used appropriately, or are there any sort of deadlines.
KK: My boss would say, go as fast as possible. That’s what I would say. We’re testing it out.
I can also recap just a couple of the main points. It really is about process engineering, which I hope through this paper, the audience can appreciate just how critical process engineering is for making chips. Because without that process engineering, the phones wouldn’t work and the chips couldn’t be built. And that’s not always really visible to most people. But the cost and time of designing those processes, those are increasing. And that’s really what this paper is trying to address. I also wanted to emphasise that this problem that we’re talking about, this process engineering problem, although Lam is interested in it, as with our equipment, it’s not really just a problem for Lam. It is a problem for our whole industry. And our role in it is that we’re just super laser-focused on the process engineering so that our equipment could work for the chip manufacturers. And then lastly, emphasise again that the human engineers continue to be essential for the chip making and for the creation of semiconductor chips. Although this hybrid approach does alleviate some of the more tedious aspects of process engineering.
And as we talked about, while the AI application to the process engineering, it’s still in its infancy, we do think the results point us to a path to foundationally change the way processes are developed in semiconductor chips.
PA: One thought occurred to me, and again, it might be a non-starter, but the way the industry is moving so that where we might characterise it, perhaps unfairly, but where there were slightly smaller amounts of process, there were mobile phones, computer chips and stuff. But the suggestion is that the future of the semiconductor is many more, but small, if you like smaller sized markets. So will this have a particular role to play there? Because rather than designing something that you’re going to bang out vast amounts of chips for a mobile phone or whatever, you’re going to have relatively shorter runs. Therefore, the process engineering development is even more important that you
can optimise or minimise the cost of that. Is that fair to say?
KK: Yeah. So I would say anywhere that you need a process engineer, that this has the potential to impact and provide cost savings and help develop that process just that much faster and that much less cost. So I think what we found even in this process here, we had the high aspect ratio, plasma etch case study that wasn’t the most complicated etch out there and we saw that it applied to that etch.
So I do think that there’s plenty of room for it to apply to kind of the more day to day process engineering for wherever process engineering is needed. This point, if you go to too difficult of a process, you need to make sure that the human can direct the computer. Because if the human can’t even figure out where to go, it’s not clear the computer will either.
Keren Kanarik, Technical Managing Director in the Office of the CTO at Lam Research