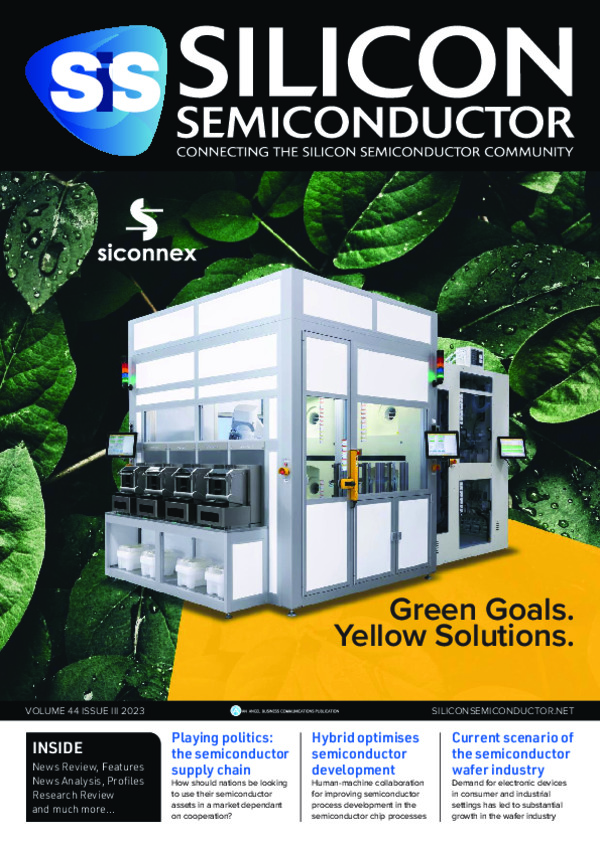
The potential of AI
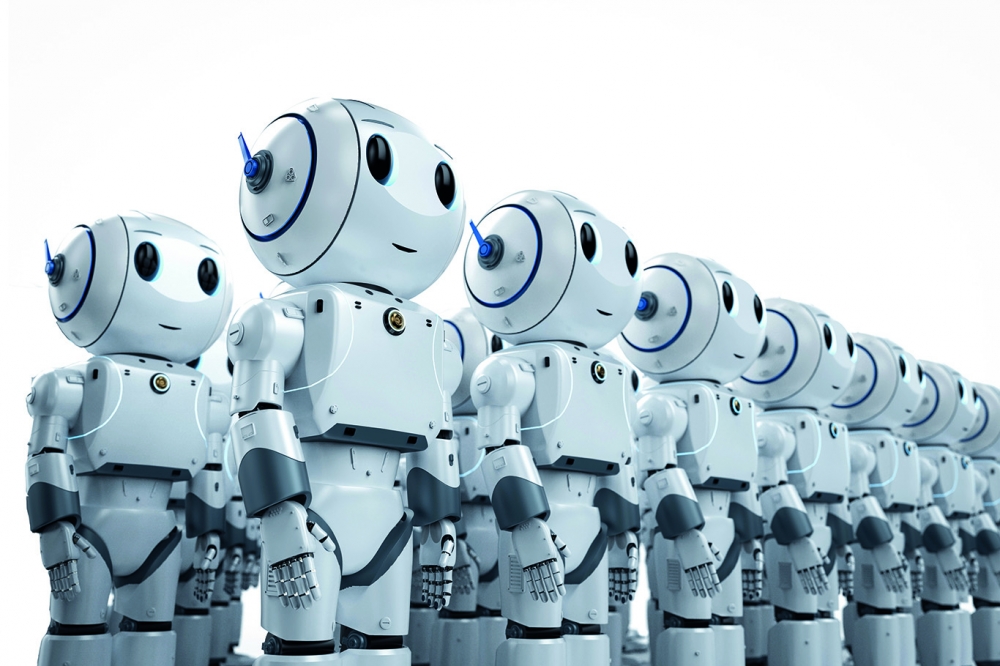
Realising impact for businesses and consumers.
By Leo Charlton (research interests are in quantum technologies and nanophotonics) is a Technology Analyst with IDTechEx.
The emergence of generative AI over the past five years – the most famous examples of which being OpenAI’s DALL-E 2 image generator and ChatGPT – has been a key milestone for the ongoing AI boom.
The robust predictive abilities of ChatGPT in particular have given a glimpse into the transformational power of AI across numerous industry verticals, where companies will be faced with the dilemma of how to effectively utilize AI tools for maximum business impact as the breadth and depth of AI models grow.
While by and large software has received more media attention of late than hardware – a not unnatural occurrence, given end-users and those that analyse the impact of such technologies care ultimately about what a tool can do, not how it can do it – the promise of AI models would remain unrealized were it not for the design and manufacture of hardware that can run these models in a cost-effective manner. As software develops in complexity (the most advanced AI models being more computationally intensive than those from yesteryear), advanced hardware is needed in order to facilitate growth.
According to a recently published report by IDTechEx on AI chips – the semiconductor circuitry that enables such AI functionalities as natural language processing, object detection and classification, and speech recognition – the global AI chips market will grow to more than US$250 billion by 2033, with the IT & Telecoms, Banking, Financial Services and Insurance (BFSI), and Consumer Electronics industries being key beneficiaries of emerging AI technologies.
Growing AI usage in edge devices
In the aforementioned AI Chips: 2023 – 2033 report, IDTechEx consider recent trends in investments for AI hardware at both the design and manufacture phase of the supply chain.
In addition to new product launches and financial data from the key market players – and model revenue growth for AI chips over the next ten years via several degrees of granularity.
A key finding from the report is related to the revenue split over the forecast period between chips used for inference purposes versus those used for training.
Training and inference are the two stages of the machine learning process, wherein computer programs utilize data to make predictions based on a model, and then optimize the model to better fit with the data provided by adjusting the weightings used. The first stage of implementing an AI algorithm is the training stage, where data is fed into the model and the model adjusts its weights until it fits appropriately with the provided data.
The second stage is the inference stage, where the trained AI algorithm is executed, and new data (that was not provided in the training stage) is classified in a manner consistent with the acquired data. Of the two stages, the training stage is more computationally intensive, given that this stage involves performing the same computation millions of times.
The training for some leading AI algorithms can take days to complete, with ChatGPT using around 10,000 Nvidia A100 GPUs to train the GPT-3.5 large language model (LLM) on which it is based. Yet, despite these already impressive numbers, IDTechEx forecast that chips used for inference purposes will grow to contribute to more than two-thirds of total AI chip market revenue as of 2033.
As all AI training takes place within the data centre in a cloud computing environment, this speaks to not only the continued use of inference chips in a cloud environment, but also the higher growth rate of AI chips used in edge devices than in a cloud environment (given that AI chips within edge devices are used for inference purposes) over the next ten years.
Adoption of AI-capable chips within edge devices is imperative to certain applications – such as fully-autonomous vehicles – and increasingly commonplace in others (such as in mobile phones). However crucial AI is to a particular application, effective deployment has the potential to create ‘new normals’ across industries.
The BFSI, Consumer Electronics, and IT & Telecoms industry verticals
are forecast to lead the way in terms of revenue generated by the sale
of AI chips up to 2033. Source: IDTechEx.
The transformative powers of AI
While the birth of ChatGPT in 2022 delivered the most compelling example to date of what generative AI is capable of, many years of development – into this and other tools– preceded it. Google DeepMind’s AlphaGo victory over Go world champion Lee Sedol in 2016 could feasibly be argued to be the AI landmark that kicked off the current boom, as it was generally considered prior to this that Go was just too difficult a game for AI to achieve victory within the time constraints of a tournament game.
IDTechEx take the view that this latest epoch in artificial intelligence began slightly earlier, with the introduction of the Siri virtual assistant to Apple phones in 2011. Siri is a virtual assistant that uses speech recognition to answer queries or follow directions from the user. By triggering the virtual assistant with the words ‘Hey Siri’, users speak into the phone, the speech recognition software translates what is spoken into computer code, and outputs text and/or a voice response from Siri. Siri’s capabilities have been expanded over the years, from simple phone commands such as ‘read my new messages’, to handling payments through Apple Pay.
While the first instance of a virtual assistant, it is not the only one, with Microsoft Cortana and Amazon’s Alexa also now widely known. Voice assistants effectively showcased the early potential of AI as applied to consumer electronic devices, where they were (and are) able to provide hands-free control and a greater range of accessibility options for users.
Since then, AI has been deployed across several different areas within consumer electronic devices to improve the user experience. Personalized recommendations are given through smart TVs and music platforms via the analysis of consumer behavior, allowing both the end-user to receive an experience tailor-made to their own tastes, while also increasing advertiser revenue.
The differing characteristics between AI at the edge and AI in the
cloud. An edge computing environment is one in which computations are
performed on a device – usually the same device on which the data is
created – that is at the edge of the network (and, therefore, close to
the user). This contrasts with cloud or data center computing, which is
at the center of the network. Source: IDTechEx.
In recent years, AI-capable smartphone chipsets have become fairly ubiquitous in leading products of major smartphone brands.
The image and object detection capabilities of these chipsets has enabled a more robust approach to photography and video on mobile phones, where camera settings are automatically adjusted according to objects in frame, and objects are able to be deleted/adjusted in post.
In the Banking, Financial Services, and Insurance industries, AI is already being put to effective use in high-frequency trading; GPU’s currently account for the majority of the market for cloud AI, where the ability to parallel process allows for the handling of large amounts of data with effective latency masking (where stalled threads are switched for threads that have data, so that computation occurs concurrently).
The hardware capabilities have enabled fraud detection in high-frequency trading, where large volumes of financial transactions are analyzed for patterns that could indicate fraudulent activities. In addition, chatbots and virtual assistants are being used across industries (not just BFSI) at the customer end, where they handle initial enquiries and automate routine tasks, improving operational efficiency for the companies that utilize these tools.
These latter examples show that the benefits of AI to companies and consumers are not mutually exclusive; personalized recommendations enhance the user experience while also bolstering company revenue via more effective marketing. In another vein, the use of AI to streamline processes via automation (as well as assist with product design based on user feedback and the analysis of potentially unstructured data) represents long-term cost-savings for companies that can identify areas of their business that can benefit from automation, and thereby free employees up for more high-value tasks. The cost savings can then have a downstream effect on product price points.
IDTechEx envision that the next ten years will see widespread AI implementation, given the fast-paced nature of developments in software and hardware, and the potential that is yet to be unlocked by the effective use of AI tools at the operational level within most companies.
AI chip applications. Source: IDTechEx